Google’s Search Tool Helps Users to Identify AI-Generated Fakes
Labeling AI-Generated Images on Facebook, Instagram and Threads Meta

This was in part to ensure that young girls were aware that models or skin didn’t look this flawless without the help of retouching. And while AI models are generally good at creating realistic-looking faces, they are less adept at hands. An extra finger or a missing limb does not automatically imply an image is fake. This is mostly because the illumination is consistently maintained and there are no issues of excessive or insufficient brightness on the rotary milking machine. The videos taken at Farm A throughout certain parts of the morning and evening have too bright and inadequate illumination as in Fig.
If content created by a human is falsely flagged as AI-generated, it can seriously damage a person’s reputation and career, causing them to get kicked out of school or lose work opportunities. And if a tool mistakes AI-generated material as real, it can go completely unchecked, potentially allowing misleading or otherwise harmful information to spread. While AI detection has been heralded by many as one way to mitigate the harms of AI-fueled misinformation and fraud, it is still a relatively new field, so results aren’t always accurate. These tools might not catch every instance of AI-generated material, and may produce false positives. These tools don’t interpret or process what’s actually depicted in the images themselves, such as faces, objects or scenes.
Although these strategies were sufficient in the past, the current agricultural environment requires a more refined and advanced approach. Traditional approaches are plagued by inherent limitations, including the need for extensive manual effort, the possibility of inaccuracies, and the potential for inducing stress in animals11. I was in a hotel room in Switzerland when I got the email, on the last international plane trip I would take for a while because I was six months pregnant. It was the end of a long day and I was tired but the email gave me a jolt. Spotting AI imagery based on a picture’s image content rather than its accompanying metadata is significantly more difficult and would typically require the use of more AI. This particular report does not indicate whether Google intends to implement such a feature in Google Photos.
How to identify AI-generated images – Mashable
How to identify AI-generated images.
Posted: Mon, 26 Aug 2024 07:00:00 GMT [source]
Photo-realistic images created by the built-in Meta AI assistant are already automatically labeled as such, using visible and invisible markers, we’re told. It’s the high-quality AI-made stuff that’s submitted from the outside that also needs to be detected in some way and marked up as such in the Facebook giant’s empire of apps. As AI-powered tools like Image Creator by Designer, ChatGPT, and DALL-E 3 become more sophisticated, identifying AI-generated content is now more difficult. The image generation tools are more advanced than ever and are on the brink of claiming jobs from interior design and architecture professionals.
But we’ll continue to watch and learn, and we’ll keep our approach under review as we do. Clegg said engineers at Meta are right now developing tools to tag photo-realistic AI-made content with the caption, “Imagined with AI,” on its apps, and will show this label as necessary over the coming months. However, OpenAI might finally have a solution for this issue (via The Decoder).
Most of the results provided by AI detection tools give either a confidence interval or probabilistic determination (e.g. 85% human), whereas others only give a binary “yes/no” result. It can be challenging to interpret these results without knowing more about the detection model, such as what it was trained to detect, the dataset used for training, and when it was last updated. Unfortunately, most online detection tools do not provide sufficient information about their development, making it difficult to evaluate and trust the detector results and their significance. AI detection tools provide results that require informed interpretation, and this can easily mislead users.
Video Detection
Image recognition is used to perform many machine-based visual tasks, such as labeling the content of images with meta tags, performing image content search and guiding autonomous robots, self-driving cars and accident-avoidance systems. Typically, image recognition entails building deep neural networks that analyze each image pixel. These networks are fed as many labeled images as possible to train them to recognize related images. Trained on data from thousands of images and sometimes boosted with information from a patient’s medical record, AI tools can tap into a larger database of knowledge than any human can. AI can scan deeper into an image and pick up on properties and nuances among cells that the human eye cannot detect. When it comes time to highlight a lesion, the AI images are precisely marked — often using different colors to point out different levels of abnormalities such as extreme cell density, tissue calcification, and shape distortions.
We are working on programs to allow us to usemachine learning to help identify, localize, and visualize marine mammal communication. Google says the digital watermark is designed to help individuals and companies identify whether an image has been created by AI tools or not. This could help people recognize inauthentic pictures published online and also protect copyright-protected images. “We’ll require people to use this disclosure and label tool when they post organic content with a photo-realistic video or realistic-sounding audio that was digitally created or altered, and we may apply penalties if they fail to do so,” Clegg said. In the long term, Meta intends to use classifiers that can automatically discern whether material was made by a neural network or not, thus avoiding this reliance on user-submitted labeling and generators including supported markings. This need for users to ‘fess up when they use faked media – if they’re even aware it is faked – as well as relying on outside apps to correctly label stuff as computer-made without that being stripped away by people is, as they say in software engineering, brittle.
The photographic record through the embedded smartphone camera and the interpretation or processing of images is the focus of most of the currently existing applications (Mendes et al., 2020). In particular, agricultural apps deploy computer vision systems to support decision-making at the crop system level, for protection and diagnosis, nutrition and irrigation, canopy management and harvest. In order to effectively track the movement of cattle, we have developed a customized algorithm that utilizes either top-bottom or left-right bounding box coordinates.
Google’s “About this Image” tool
The AMI systems also allow researchers to monitor changes in biodiversity over time, including increases and decreases. Researchers have estimated that globally, due to human activity, species are going extinct between 100 and 1,000 times faster than they usually would, so monitoring wildlife is vital to conservation efforts. The researchers blamed that in part on the low resolution of the images, which came from a public database.
- The biggest threat brought by audiovisual generative AI is that it has opened up the possibility of plausible deniability, by which anything can be claimed to be a deepfake.
- AI proposes important contributions to knowledge pattern classification as well as model identification that might solve issues in the agricultural domain (Lezoche et al., 2020).
- Moreover, the effectiveness of Approach A extends to other datasets, as reflected in its better performance on additional datasets.
- In GranoScan, the authorization filter has been implemented following OAuth2.0-like specifications to guarantee a high-level security standard.
Developed by scientists in China, the proposed approach uses mathematical morphologies for image processing, such as image enhancement, sharpening, filtering, and closing operations. It also uses image histogram equalization and edge detection, among other methods, to find the soiled spot. Katriona Goldmann, a research data scientist at The Alan Turing Institute, is working with Lawson to train models to identify animals recorded by the AMI systems. Similar to Badirli’s 2023 study, Goldmann is using images from public databases. Her models will then alert the researchers to animals that don’t appear on those databases. This strategy, called “few-shot learning” is an important capability because new AI technology is being created every day, so detection programs must be agile enough to adapt with minimal training.
Recent Artificial Intelligence Articles
With this method, paper can be held up to a light to see if a watermark exists and the document is authentic. “We will ensure that every one of our AI-generated images has a markup in the original file to give you context if you come across it outside of our platforms,” Dunton said. He added that several image publishers including Shutterstock and Midjourney would launch similar labels in the coming months. Our Community Standards apply to all content posted on our platforms regardless of how it is created.
- Where \(\theta\)\(\rightarrow\) parameters of the autoencoder, \(p_k\)\(\rightarrow\) the input image in the dataset, and \(q_k\)\(\rightarrow\) the reconstructed image produced by the autoencoder.
- Livestock monitoring techniques mostly utilize digital instruments for monitoring lameness, rumination, mounting, and breeding.
- These results represent the versatility and reliability of Approach A across different data sources.
- This was in part to ensure that young girls were aware that models or skin didn’t look this flawless without the help of retouching.
- The AMI systems also allow researchers to monitor changes in biodiversity over time, including increases and decreases.
This has led to the emergence of a new field known as AI detection, which focuses on differentiating between human-made and machine-produced creations. With the rise of generative AI, it’s easy and inexpensive to make highly convincing fabricated content. Today, artificial content and image generators, as well as deepfake technology, are used in all kinds of ways — from students taking shortcuts on their homework to fraudsters disseminating false information about wars, political elections and natural disasters. However, in 2023, it had to end a program that attempted to identify AI-written text because the AI text classifier consistently had low accuracy.
A US agtech start-up has developed AI-powered technology that could significantly simplify cattle management while removing the need for physical trackers such as ear tags. “Using our glasses, we were able to identify dozens of people, including Harvard students, without them ever knowing,” said Ardayfio. After a user inputs media, Winston AI breaks down the probability the text is AI-generated and highlights the sentences it suspects were written with AI. Akshay Kumar is a veteran tech journalist with an interest in everything digital, space, and nature. Passionate about gadgets, he has previously contributed to several esteemed tech publications like 91mobiles, PriceBaba, and Gizbot. Whenever he is not destroying the keyboard writing articles, you can find him playing competitive multiplayer games like Counter-Strike and Call of Duty.
iOS 18 hits 68% adoption across iPhones, per new Apple figures
The project identified interesting trends in model performance — particularly in relation to scaling. Larger models showed considerable improvement on simpler images but made less progress on more challenging images. The CLIP models, which incorporate both language and vision, stood out as they moved in the direction of more human-like recognition.
The original decision layers of these weak models were removed, and a new decision layer was added, using the concatenated outputs of the two weak models as input. This new decision layer was trained and validated on the same training, validation, and test sets while keeping the convolutional layers from the original weak models frozen. Lastly, a fine-tuning process was applied to the entire ensemble model to achieve optimal results. The datasets were then annotated and conditioned in a task-specific fashion. In particular, in tasks related to pests, weeds and root diseases, for which a deep learning model based on image classification is used, all the images have been cropped to produce square images and then resized to 512×512 pixels. Images were then divided into subfolders corresponding to the classes reported in Table1.
The remaining study is structured into four sections, each offering a detailed examination of the research process and outcomes. Section 2 details the research methodology, encompassing dataset description, image segmentation, feature extraction, and PCOS classification. Subsequently, Section 3 conducts a thorough analysis of experimental results. Finally, Section 4 encapsulates the key findings of the study and outlines potential future research directions.
When it comes to harmful content, the most important thing is that we are able to catch it and take action regardless of whether or not it has been generated using AI. And the use of AI in our integrity systems is a big part of what makes it possible for us to catch it. In the meantime, it’s important people consider several things when determining if content has been created by AI, like checking whether the account sharing the content is trustworthy or looking for details that might look or sound unnatural. “Ninety nine point nine percent of the time they get it right,” Farid says of trusted news organizations.
These tools are trained on using specific datasets, including pairs of verified and synthetic content, to categorize media with varying degrees of certainty as either real or AI-generated. The accuracy of a tool depends on the quality, quantity, and type of training data used, as well as the algorithmic functions that it was designed for. For instance, a detection model may be able to spot AI-generated images, but may not be able to identify that a video is a deepfake created from swapping people’s faces.
To address this issue, we resolved it by implementing a threshold that is determined by the frequency of the most commonly predicted ID (RANK1). If the count drops below a pre-established threshold, we do a more detailed examination of the RANK2 data to identify another potential ID that occurs frequently. The cattle are identified as unknown only if both RANK1 and RANK2 do not match the threshold. Otherwise, the most frequent ID (either RANK1 or RANK2) is issued to ensure reliable identification for known cattle. We utilized the powerful combination of VGG16 and SVM to completely recognize and identify individual cattle. VGG16 operates as a feature extractor, systematically identifying unique characteristics from each cattle image.
Image recognition accuracy: An unseen challenge confounding today’s AI
“But for AI detection for images, due to the pixel-like patterns, those still exist, even as the models continue to get better.” Kvitnitsky claims AI or Not achieves a 98 percent accuracy rate on average. Meanwhile, Apple’s upcoming Apple Intelligence features, which let users create new emoji, edit photos and create images using AI, are expected to add code to each image for easier AI identification. Google is planning to roll out new features that will enable the identification of images that have been generated or edited using AI in search results.

These annotations are then used to create machine learning models to generate new detections in an active learning process. While companies are starting to include signals in their image generators, they haven’t started including them in AI tools that generate audio and video at the same scale, so we can’t yet detect those signals and label this content from other companies. While the industry works towards this capability, we’re adding a feature for people to disclose when they share AI-generated video or audio so we can add a label to it. We’ll require people to use this disclosure and label tool when they post organic content with a photorealistic video or realistic-sounding audio that was digitally created or altered, and we may apply penalties if they fail to do so.
Detection tools should be used with caution and skepticism, and it is always important to research and understand how a tool was developed, but this information may be difficult to obtain. The biggest threat brought by audiovisual generative AI is that it has opened up the possibility of plausible deniability, by which anything can be claimed to be a deepfake. With the progress of generative AI technologies, synthetic media is getting more realistic.
This is found by clicking on the three dots icon in the upper right corner of an image. AI or Not gives a simple “yes” or “no” unlike other AI image detectors, but it correctly said the image was AI-generated. Other AI detectors that have generally high success rates include Hive Moderation, SDXL Detector on Hugging Face, and Illuminarty.
Discover content
Common object detection techniques include Faster Region-based Convolutional Neural Network (R-CNN) and You Only Look Once (YOLO), Version 3. R-CNN belongs to a family of machine learning models for computer vision, specifically object detection, whereas YOLO is a well-known real-time object detection algorithm. The training and validation process for the ensemble model involved dividing each dataset into training, testing, and validation sets with an 80–10-10 ratio. Specifically, we began with end-to-end training of multiple models, using EfficientNet-b0 as the base architecture and leveraging transfer learning. Each model was produced from a training run with various combinations of hyperparameters, such as seed, regularization, interpolation, and learning rate. From the models generated in this way, we selected the two with the highest F1 scores across the test, validation, and training sets to act as the weak models for the ensemble.
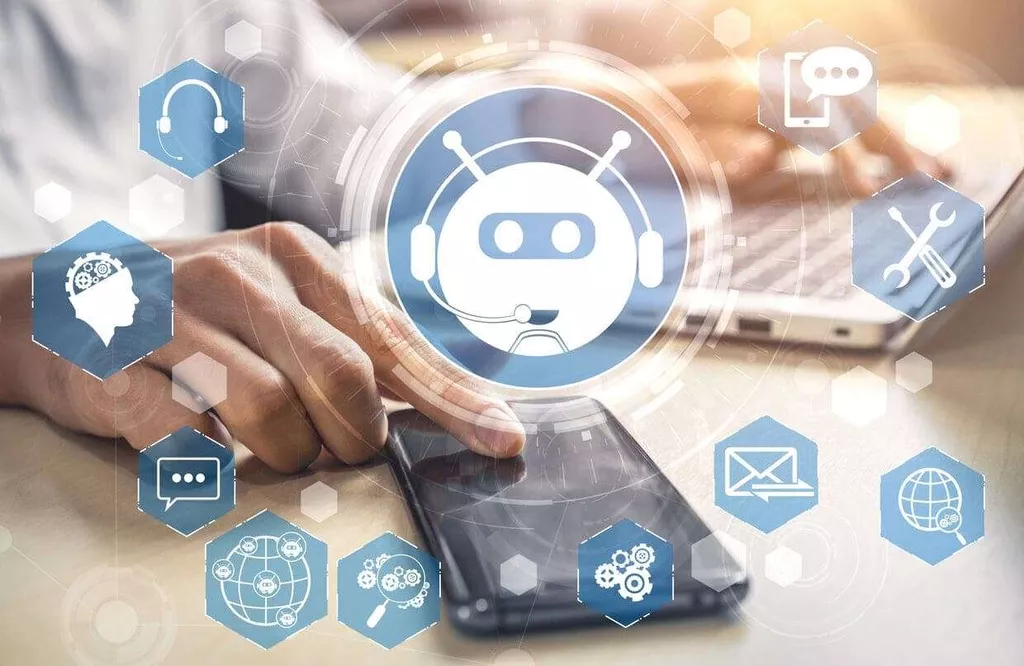
In this system, the ID-switching problem was solved by taking the consideration of the number of max predicted ID from the system. The collected cattle images which were grouped by their ground-truth ID after tracking results were used as datasets to train in the VGG16-SVM. VGG16 extracts the features from the cattle images inside the folder of each tracked cattle, which can be trained with the SVM for final identification ID. After extracting the features in the VGG16 the extracted features were trained in SVM.

On the flip side, the Starling Lab at Stanford University is working hard to authenticate real images. Starling Lab verifies “sensitive digital records, such as the documentation of human rights violations, war crimes, and testimony of genocide,” and securely stores verified digital images in decentralized networks so they can’t be tampered with. The lab’s work isn’t user-facing, but its library of projects are a good resource for someone looking to authenticate images of, say, the war in Ukraine, or the presidential transition from Donald Trump to Joe Biden. This isn’t the first time Google has rolled out ways to inform users about AI use. In July, the company announced a feature called About This Image that works with its Circle to Search for phones and in Google Lens for iOS and Android.

However, a majority of the creative briefs my clients provide do have some AI elements which can be a very efficient way to generate an initial composite for us to work from. When creating images, there’s really no use for something that doesn’t provide the exact result I’m looking for. I completely understand social media outlets needing to label potential AI images but it must be immensely frustrating for creatives when improperly applied.